Documents with DataMapPlot
¶
Visualize documents and their topics in 2D as a static plot for publication using DataMapPlot.
Parameters:
Name | Type | Description | Default |
---|---|---|---|
topic_model |
A fitted BERTopic instance. |
required | |
docs |
List[str] |
The documents you used when calling either |
required |
topics |
List[int] |
A selection of topics to visualize.
Not to be confused with the topics that you get from |
None |
embeddings |
ndarray |
The embeddings of all documents in |
None |
reduced_embeddings |
ndarray |
The 2D reduced embeddings of all documents in |
None |
custom_labels |
Union[bool, str] |
If bool, whether to use custom topic labels that were defined using
|
False |
title |
str |
Title of the plot. |
'Documents and Topics' |
sub_title |
Optional[str] |
Sub-title of the plot. |
None |
width |
int |
The width of the figure. |
1200 |
height |
int |
The height of the figure. |
1200 |
**datamap_kwds |
All further keyword args will be passed on to DataMapPlot's
|
{} |
Returns:
Type | Description |
---|---|
figure |
A Matplotlib Figure object. |
Examples:
To visualize the topics simply run:
topic_model.visualize_document_datamap(docs)
Do note that this re-calculates the embeddings and reduces them to 2D. The advised and preferred pipeline for using this function is as follows:
from sklearn.datasets import fetch_20newsgroups
from sentence_transformers import SentenceTransformer
from bertopic import BERTopic
from umap import UMAP
# Prepare embeddings
docs = fetch_20newsgroups(subset='all', remove=('headers', 'footers', 'quotes'))['data']
sentence_model = SentenceTransformer("all-MiniLM-L6-v2")
embeddings = sentence_model.encode(docs, show_progress_bar=False)
# Train BERTopic
topic_model = BERTopic().fit(docs, embeddings)
# Reduce dimensionality of embeddings, this step is optional
# reduced_embeddings = UMAP(n_neighbors=10, n_components=2, min_dist=0.0, metric='cosine').fit_transform(embeddings)
# Run the visualization with the original embeddings
topic_model.visualize_document_datamap(docs, embeddings=embeddings)
# Or, if you have reduced the original embeddings already:
topic_model.visualize_document_datamap(docs, reduced_embeddings=reduced_embeddings)
Or if you want to save the resulting figure:
fig = topic_model.visualize_document_datamap(docs, reduced_embeddings=reduced_embeddings)
fig.savefig("path/to/file.png", bbox_inches="tight")
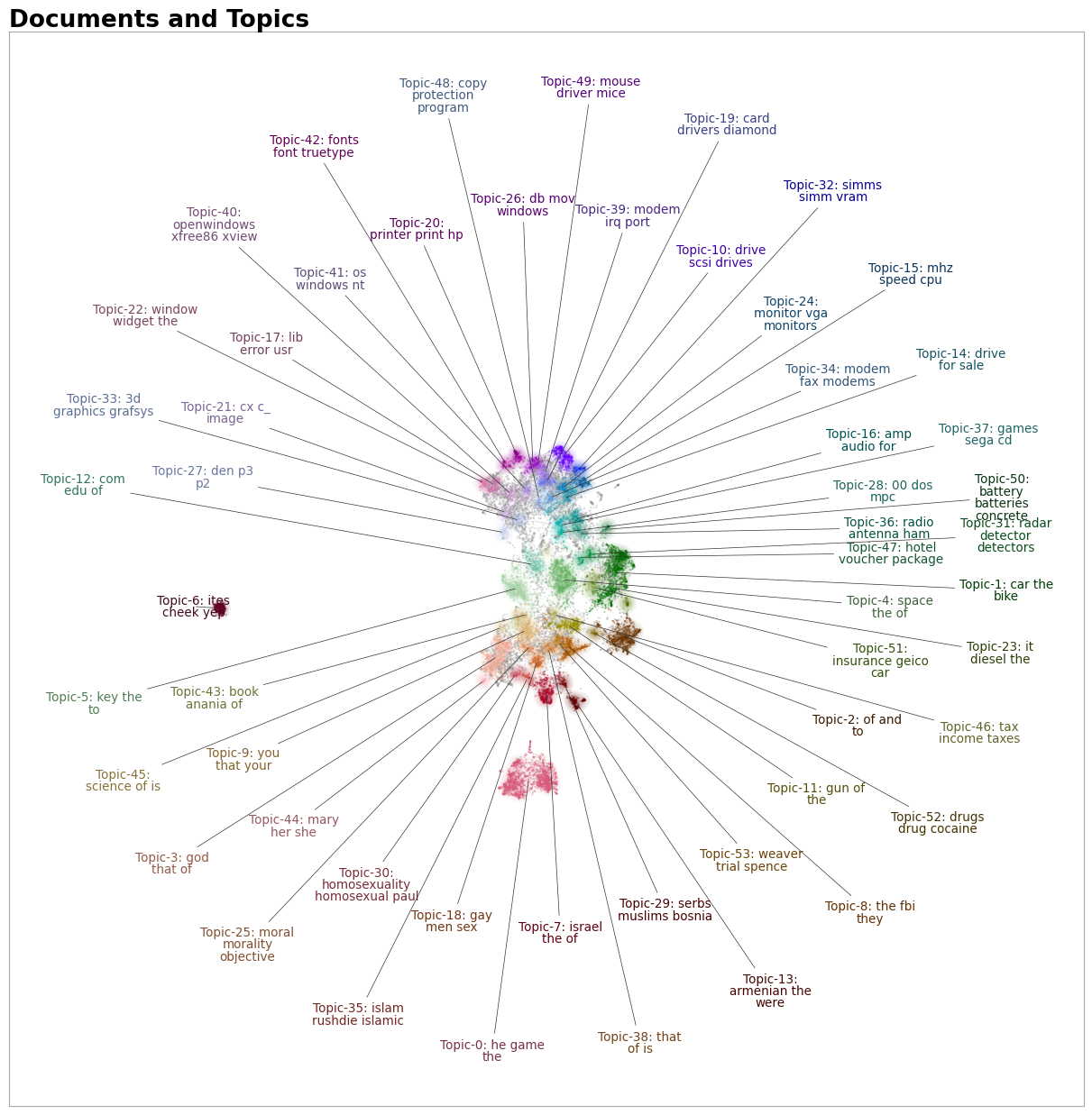
Source code in bertopic\plotting\_datamap.py
def visualize_document_datamap(
topic_model,
docs: List[str],
topics: List[int] = None,
embeddings: np.ndarray = None,
reduced_embeddings: np.ndarray = None,
custom_labels: Union[bool, str] = False,
title: str = "Documents and Topics",
sub_title: Union[str, None] = None,
width: int = 1200,
height: int = 1200,
**datamap_kwds,
) -> Figure:
"""Visualize documents and their topics in 2D as a static plot for publication using
DataMapPlot.
Arguments:
topic_model: A fitted BERTopic instance.
docs: The documents you used when calling either `fit` or `fit_transform`
topics: A selection of topics to visualize.
Not to be confused with the topics that you get from `.fit_transform`.
For example, if you want to visualize only topics 1 through 5:
`topics = [1, 2, 3, 4, 5]`. Documents not in these topics will be shown
as noise points.
embeddings: The embeddings of all documents in `docs`.
reduced_embeddings: The 2D reduced embeddings of all documents in `docs`.
custom_labels: If bool, whether to use custom topic labels that were defined using
`topic_model.set_topic_labels`.
If `str`, it uses labels from other aspects, e.g., "Aspect1".
title: Title of the plot.
sub_title: Sub-title of the plot.
width: The width of the figure.
height: The height of the figure.
**datamap_kwds: All further keyword args will be passed on to DataMapPlot's
`create_plot` function. See the DataMapPlot documentation
for more details.
Returns:
figure: A Matplotlib Figure object.
Examples:
To visualize the topics simply run:
```python
topic_model.visualize_document_datamap(docs)
```
Do note that this re-calculates the embeddings and reduces them to 2D.
The advised and preferred pipeline for using this function is as follows:
```python
from sklearn.datasets import fetch_20newsgroups
from sentence_transformers import SentenceTransformer
from bertopic import BERTopic
from umap import UMAP
# Prepare embeddings
docs = fetch_20newsgroups(subset='all', remove=('headers', 'footers', 'quotes'))['data']
sentence_model = SentenceTransformer("all-MiniLM-L6-v2")
embeddings = sentence_model.encode(docs, show_progress_bar=False)
# Train BERTopic
topic_model = BERTopic().fit(docs, embeddings)
# Reduce dimensionality of embeddings, this step is optional
# reduced_embeddings = UMAP(n_neighbors=10, n_components=2, min_dist=0.0, metric='cosine').fit_transform(embeddings)
# Run the visualization with the original embeddings
topic_model.visualize_document_datamap(docs, embeddings=embeddings)
# Or, if you have reduced the original embeddings already:
topic_model.visualize_document_datamap(docs, reduced_embeddings=reduced_embeddings)
```
Or if you want to save the resulting figure:
```python
fig = topic_model.visualize_document_datamap(docs, reduced_embeddings=reduced_embeddings)
fig.savefig("path/to/file.png", bbox_inches="tight")
```
<img src="../../getting_started/visualization/datamapplot.png",
alt="DataMapPlot of 20-Newsgroups", width=800, height=800></img>
"""
topic_per_doc = topic_model.topics_
df = pd.DataFrame({"topic": np.array(topic_per_doc)})
df["doc"] = docs
df["topic"] = topic_per_doc
# Extract embeddings if not already done
if embeddings is None and reduced_embeddings is None:
embeddings_to_reduce = topic_model._extract_embeddings(df.doc.to_list(), method="document")
else:
embeddings_to_reduce = embeddings
# Reduce input embeddings
if reduced_embeddings is None:
umap_model = UMAP(n_neighbors=15, n_components=2, min_dist=0.15, metric="cosine").fit(embeddings_to_reduce)
embeddings_2d = umap_model.embedding_
else:
embeddings_2d = reduced_embeddings
unique_topics = set(topic_per_doc)
# Prepare text and names
if isinstance(custom_labels, str):
names = [[[str(topic), None]] + topic_model.topic_aspects_[custom_labels][topic] for topic in unique_topics]
names = [" ".join([label[0] for label in labels[:4]]) for labels in names]
names = [label if len(label) < 30 else label[:27] + "..." for label in names]
elif topic_model.custom_labels_ is not None and custom_labels:
names = [topic_model.custom_labels_[topic + topic_model._outliers] for topic in unique_topics]
else:
names = [
f"Topic-{topic}: " + " ".join([word for word, value in topic_model.get_topic(topic)][:3])
for topic in unique_topics
]
topic_name_mapping = {topic_num: topic_name for topic_num, topic_name in zip(unique_topics, names)}
topic_name_mapping[-1] = "Unlabelled"
# If a set of topics is chosen, set everything else to "Unlabelled"
if topics is not None:
selected_topics = set(topics)
for topic_num in topic_name_mapping:
if topic_num not in selected_topics:
topic_name_mapping[topic_num] = "Unlabelled"
# Map in topic names and plot
named_topic_per_doc = pd.Series(topic_per_doc).map(topic_name_mapping).values
figure, axes = datamapplot.create_plot(
embeddings_2d,
named_topic_per_doc,
figsize=(width / 100, height / 100),
dpi=100,
title=title,
sub_title=sub_title,
**datamap_kwds,
)
return figure